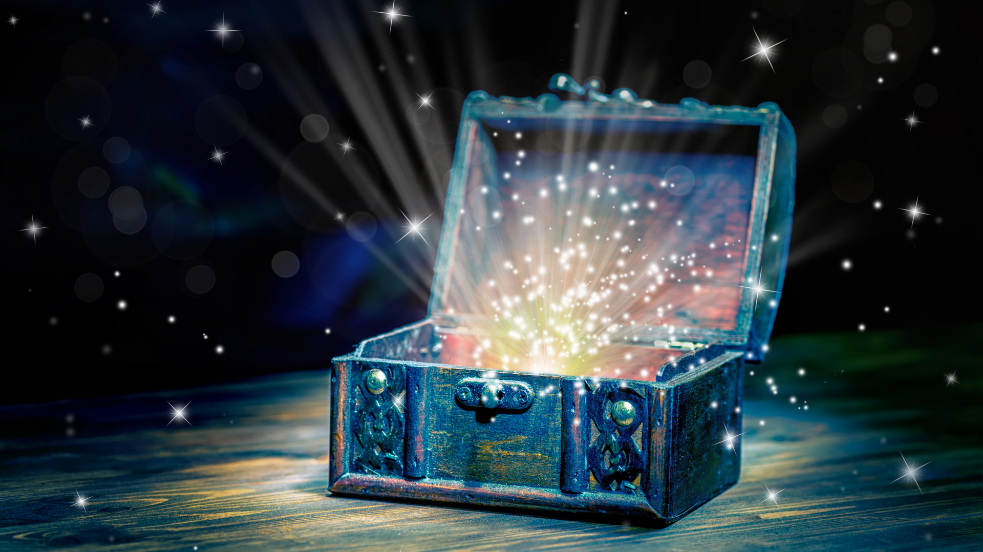
The huge potential of RPA has been seen across many data-driven industries since its inception as a technology. But for years now, practical applications have been limited.
That’s because not every piece of data that could be put to use by a robot is readily available in the most straightforward formats. Some information has been ‘locked’ in pictures or videos. Others are in handwriting, or skewed, low-resolution scans. This is called ‘dark data’, or more technically, ‘unstructured data’.
Thus, processes that by all other means fit the bill for Robotic Process Automation – e.g. repetitive & data-heavy tasks – haven’t benefited from this emerging technology… yet!
But bots have been getting more intelligent. UiPath’s latest product – the Document Understanding Framework – now enables bots to shine a light into dark data.
The Document Understanding Framework sits at the intersection of Document Processing, RPA, and AI.
It’s more powerful than traditional digitization (which uses OCR engines to convert documents into text, but leaves contents unformatted). As the name implies, the DUF understands information in the documents. Here is what the DUF enables bots to do:
- Identify – identify what data is important for the process, and where in a document that data lies.
- Interpret – pick the best OCR engines for each process’ requirements. No one OCR is best for every scenario. For example, one engine might be best for translating info from German into English, but a different engine could be better for digitizing handwritten English texts.
- Classify – understand which page(s) in multi-page documents contain the necessary info, and separate these for further use. (AKA “Package Splitting” or “Document Splitting”). Humans can remain in the loop to go through what the robot has split, but it is not necessary for them to.
- Extract – extracting data from documents and arranging it into the designated data processing application (such as XLS spreadsheet).
- Validation – capture labeled data outputs from validation stations. These can be used as training sets for ongoing ML model improvement.
The Document Understanding Framework unlocks difficult, dark data from unstructured documents, and opens up more opportunities for automation across data-driven industries.